DevOps Observability: Best Practices for Reliable Systems
Unlock system reliability with DevOps observability. Learn best practices for metrics, logging, tracing, and alerts to proactively manage your infrastructure and applications.
DevOps Observability: Best Practices for Reliable Systems
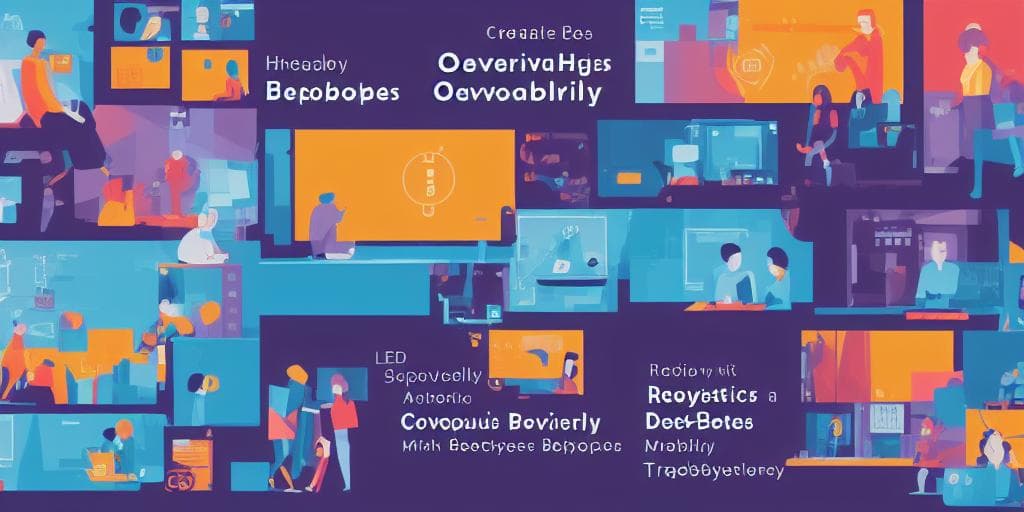
In today's fast-paced software development landscape, maintaining system reliability is paramount. DevOps teams strive to deliver value quickly and efficiently, but this agility can introduce complexity. That's where observability comes in. It's more than just monitoring; it's about understanding the internal state of your systems by examining their outputs – metrics, logs, and traces. By implementing effective observability practices, you can proactively identify and resolve issues, optimize performance, and ultimately ensure a positive user experience. This blog post will delve into the core principles of observability and provide practical best practices for integrating it into your DevOps workflow, turning data into actionable insights.
The Pillars of Observability: Metrics, Logs, and Traces
Observability rests on three fundamental pillars: metrics, logs, and traces. Understanding how each pillar contributes to a comprehensive view of your system is crucial.
* **Metrics:** These are numerical representations of system behavior collected over time. They provide aggregated insights into resource utilization, performance, and overall system health. Examples include CPU utilization, memory usage, request latency, and error rates. Effective metrics are granular, well-defined, and easy to aggregate. Using a time-series database like Prometheus allows you to efficiently store and query metric data. Tools such as Grafana can visualize these metrics, making it easier to spot trends and anomalies.
* **Logs:** Logs are records of events that occur within your system. They provide detailed context about individual transactions or operations. Logs can be structured (e.g., JSON) or unstructured (e.g., plain text). Structured logs are easier to parse and query. Centralized logging systems like the ELK stack (Elasticsearch, Logstash, Kibana) or Splunk enable you to collect, process, and analyze logs from across your infrastructure. Ensure your logs include relevant information such as timestamps, request IDs, user IDs, and error messages.
* **Traces:** Traces track the journey of a request as it propagates through different services in a distributed system. They provide insights into dependencies between services and pinpoint bottlenecks. Distributed tracing tools like Jaeger or Zipkin automatically instrument your code to capture trace data. Each trace consists of spans, which represent individual units of work. Analyzing traces helps you understand the flow of requests, identify performance bottlenecks, and diagnose errors in complex microservices architectures.
Choosing the right tools and techniques for collecting, storing, and analyzing metrics, logs, and traces is crucial for building a robust observability strategy. Each pillar provides a unique perspective on your system's behavior, and combining them provides a holistic view that enables you to proactively identify and resolve issues.
Best Practices for Each Pillar
- **Metrics:**
- Choose metrics relevant to your business goals.
- Use appropriate aggregation intervals (e.g., 1 minute, 5 minutes).
- Set up alerts based on metric thresholds.- **Metrics:**
- **Logs:**
- Use structured logging formats.
- Centralize your logs.
- Implement log rotation and retention policies.- **Logs:**
- **Traces:**
- Instrument your code with tracing libraries.
- Propagate tracing context across services.
- Analyze traces to identify performance bottlenecks.- **Traces:**
```python
# Example of logging in Python
import logging
import json
logging.basicConfig(level=logging.INFO)
logger = logging.getLogger(__name__)
def process_request(request_id, user_id):
data = {
'request_id': request_id,
'user_id': user_id,
'message': 'Request processing started'
}
logger.info(json.dumps(data))
```
Implementing Observability in Your DevOps Pipeline
Observability should be integrated into every stage of your DevOps pipeline, from development to production. Shifting observability left ensures that issues are identified and addressed early, reducing the risk of problems in production.
* **Development:** Developers should use logging and tracing tools to instrument their code and gain insights into its behavior. Unit tests and integration tests should include assertions about the expected output of metrics, logs, and traces. Tools like OpenTelemetry provide a vendor-neutral API for instrumenting code for observability.
* **Testing:** Performance testing and load testing should be used to identify performance bottlenecks and ensure that your system can handle the expected load. Monitoring metrics, logs, and traces during testing helps you understand how your system behaves under stress and identify areas for optimization.
* **Deployment:** Automated deployment pipelines should include checks for observability before and after deploying new code. This helps you ensure that the new code is behaving as expected and that no new issues have been introduced. Canary deployments and blue-green deployments can be used to gradually roll out new code and monitor its impact on system performance and stability.
* **Production:** Continuous monitoring of metrics, logs, and traces is essential for identifying and resolving issues in production. Automated alerts should be configured to notify you of potential problems before they impact users. Incident response plans should include procedures for using observability data to diagnose and resolve issues quickly.
By integrating observability into your DevOps pipeline, you can improve the reliability, performance, and security of your systems. This shift-left approach to observability empowers developers to build more resilient applications and enables operations teams to proactively manage their infrastructure.
```java
// Example of using OpenTelemetry in Java
import io.opentelemetry.api.GlobalOpenTelemetry;
import io.opentelemetry.api.trace.Span;
import io.opentelemetry.api.trace.Tracer;
public class MyService {
private static final Tracer tracer = GlobalOpenTelemetry.getTracer("MyService", "1.0.0");
public void doSomething() {
Span span = tracer.spanBuilder("doSomething").startSpan();
try {
// Your code here
} finally {
span.end();
}
}
}
```
Alerting and Automated Remediation
Observability data is only valuable if it leads to timely action. Alerting is the process of notifying you when something goes wrong. Automated remediation is the process of automatically taking action to resolve issues.
* **Alerting:** Alerts should be based on well-defined thresholds and should be tailored to the specific needs of your application. Avoid creating too many alerts, as this can lead to alert fatigue. Focus on creating alerts that are actionable and that provide clear guidance on how to resolve the issue. Tools like Prometheus Alertmanager can be used to manage alerts and route them to the appropriate team.
* **Automated Remediation:** Automated remediation can be used to automatically resolve common issues, such as restarting a service or scaling up resources. This can help reduce the time it takes to resolve issues and improve system availability. Tools like Ansible, Terraform, and Kubernetes can be used to automate remediation tasks. However, it's important to carefully design and test automated remediation procedures to ensure that they don't inadvertently cause more problems.
By combining alerting and automated remediation, you can create a self-healing system that is resilient to failures. This reduces the burden on your operations team and allows them to focus on more strategic initiatives. When setting up alerts, consider these levels of alerting:
- 01.
- **Informational:** These alerts provide insights into system behavior, but do not necessarily indicate a problem.
- 02.
- **Warning:** These alerts indicate that a problem may be developing.
- 03.
- **Critical:** These alerts indicate that a problem is actively impacting users.
Automating incident response can significantly decrease downtime and improve overall system stability. Ensure proper logging of automated actions for audit and analysis.
```yaml
# Example Prometheus Alertmanager configuration
route:
receiver: 'slack-notifications'
inhibit_rules:
- source_match:
severity: 'critical'
target_match:
severity: 'warning'
equal: ['alertname', 'dev', 'instance']
receivers:
- name: 'slack-notifications'
slack_configs:
- api_url: '$SLACK_WEBHOOK_URL'
channel: '#alerts'
send_resolved: true
```
Conclusion
Embracing observability is not just a technical implementation; it's a cultural shift towards a more proactive and data-driven approach to DevOps. By focusing on metrics, logs, and traces, and integrating these practices throughout your pipeline, you empower your teams to build and maintain more reliable and resilient systems. As a next step, evaluate your current monitoring practices and identify areas where you can improve observability. Explore different tools and technologies, experiment with automated remediation, and continuously refine your strategy based on the insights you gain. Investing in observability will ultimately translate to improved system uptime, faster incident resolution, and happier users.
packages
build Easily by using less dependent On Others Use Our packages , Robust and Long term support
Help Your Friend By Sharing the Packages
Do You Want to Discuss About Your Idea ?